by Janne-Pekka Karttunen
CEO, Distence
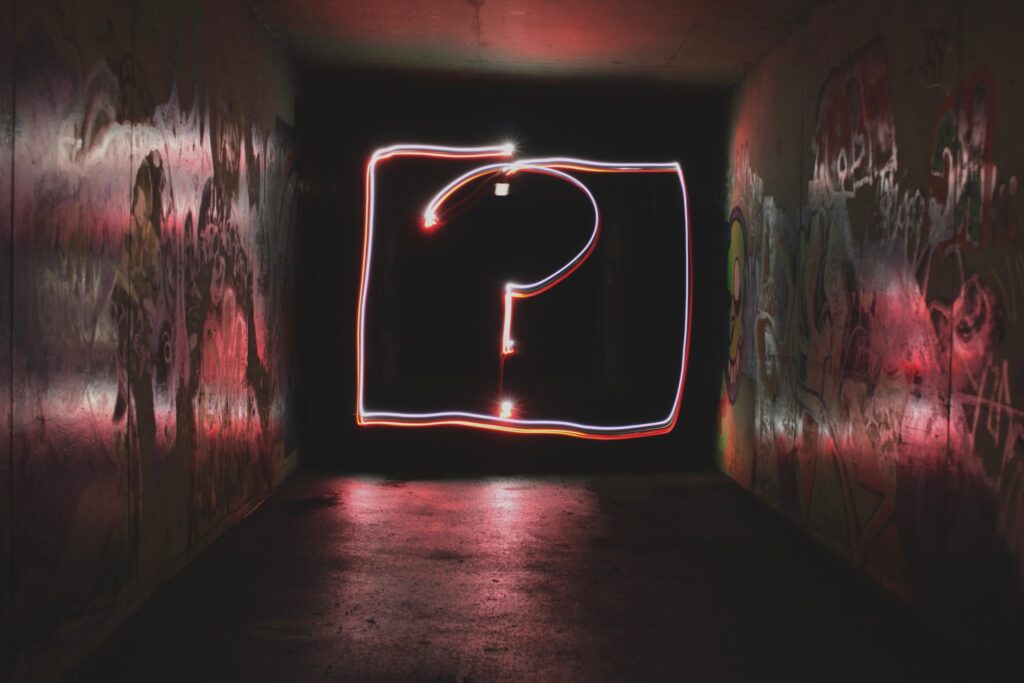
While defining the right problem for the traditional study it is vital for Machine learning to have quality data to build on. I have recently heard several times the phrase “your AI is as good as your data”. In predictive maintenance, data often originates from process controllers, designed to optimize the industrial process; therefore, it might not be optimal for predictive maintenance? – McKinsey published an excellent article on this topic, titled “Predictive maintenance: the wrong solution to the right problem in chemicals“.
The authors list four main reasons to justify the title and explain why poor results are delivered: 1) Too little data or too few data points for the system to learn from. 2) Too short time to react; when a prediction is given two days beforehand the preparation of maintenance, which might require a month or more, it provides simply too little time to react. 3) Too little financial impact as processes are planned to be redundant, and 4) Too small savings as the majority of downtime cost comes from planned maintenance. The study considers the chemical industry. While we at Distence can align with many of the arguments, we also see them applicable in many other manufacturing industries.
The first argument raises the point that many professionals are starting to note in public. Mr Tokohimo from Lingköping university noted that “One big hurdle is that the data needs to be collected and stored in a way that allows AI to be applied.“, while David Bell from Ge Digital shared a similar observation in late 2019, “A big challenge in the industrial analytics world is that most machine learning and AI techniques are data-driven. While the industrial world has a lot of data, the type of data needed for analysis from a reliability and maintenance perspective is hard to access because these systems were not designed for this type of analysis“. This is why we at Distence created an open and data-driven platform Condence for the rotating machinery market.
The second argument might originate from the various reasons for failure and from the fact that many of the failures seen in the process data are already “functional failures” which by default lead to short lead times. If, however, the monitoring system is designed for the purpose, in many cases, mechanical failures can be seen months or even a year in advance. For the system to reach this level of accuracy, it is vital that the driver here is again that quality of data and understanding the physics of the problem.
Argument number three is easy to agree within the sense that when the approach is “run to failure” or the financial impact is only considered given the second argument on short lead time. However, I would like to note that if two days would be two months and the data is used to avoid the problem or perform the necessary repairs without a planned maintenance shutdown, avoiding the extra downtime, the financial impact would be substantially higher.
The fourth argument is also very valid in the context of the study. As the McKinsey researchers note later, it is not only converting the unplanned to planned maintenance but to allocate the CAPEX investments as well as operational activities in the most efficient way.
All of the arguments support the fact that the digital journey begins with quality data and applying that right. For rotating machinery, we believe that there is not enough data to build reliable statistical systems delivering long lead times. However, one study made by one of our customers optimized systems on top of the quality data system detected problems in 80% cases before professional’s had caught it in the real situation.
There is a growing need for high accuracy data, stored and labelled in the way that it can be post-processed by machine learning and AI. This type of data necessitates purpose-built metering and systems. This is how we can deliver true value for predictive maintenance with machine learning.